Code
library(dlookr)
The dlookr is a collection of tools that support data diagnosis, exploration, and transformation. Data diagnostics provides information and visualization of missing values and outliers and unique and negative values to help you understand the distribution and quality of your data. Data exploration provides information and visualization of the descriptive statistics of univariate variables, normality tests and outliers, correlation of two variables, and relationship between target variable and predictor. Data transformation supports binning for categorizing continuous variables, imputates missing values and outliers, resolving skewness. And it creates automated reports that support these three tasks.
Features:
Diagnose data quality.
Find appropriate scenarios to pursuit the follow-up analysis through data exploration and understanding.
Derive new variables or perform variable transformations.
Automatically generate reports for the above three tasks.
Supports quality diagnosis and EDA of table of DBMS
Data quality diagnosis for data.frame, tbl_df, and table of DBMS
Exploratory Data Analysis for data.frame, tbl_df, and table of DBMS
Data Transformation
Data diagnosis and EDA for table of DBMS
install.packages("dlookr")
In this exercise we will use following data set. The data set has missing values.
Data Quality Diagnosis is the first step before any statistical analysis. We use diagnose() function of dlookr package to do general General diagnosis of all variables.
The variables of the tbl_df object returned by diagnose () are as follows:
variables : variable names
types : the data type of the variables
missing_count : number of missing values
missing_percent : percentage of missing values
unique_count : number of unique values
unique_rate : rate of unique value. unique_count / number of observation
variables | types | missing_count | missing_percent | unique_count | unique_rate |
---|---|---|---|---|---|
ID | numeric | 0 | 0.0000000 | 471 | 1.000000000 |
FIPS | numeric | 0 | 0.0000000 | 172 | 0.365180467 |
STATE_ID | numeric | 0 | 0.0000000 | 4 | 0.008492569 |
STATE | character | 0 | 0.0000000 | 4 | 0.008492569 |
COUNTY | character | 0 | 0.0000000 | 161 | 0.341825902 |
Longitude | numeric | 0 | 0.0000000 | 471 | 1.000000000 |
Latitude | numeric | 0 | 0.0000000 | 471 | 1.000000000 |
SOC | numeric | 4 | 0.8492569 | 457 | 0.970276008 |
DEM | numeric | 0 | 0.0000000 | 464 | 0.985138004 |
Aspect | numeric | 0 | 0.0000000 | 464 | 0.985138004 |
Slope | numeric | 0 | 0.0000000 | 464 | 0.985138004 |
TPI | numeric | 0 | 0.0000000 | 464 | 0.985138004 |
KFactor | numeric | 0 | 0.0000000 | 386 | 0.819532909 |
MAP | numeric | 0 | 0.0000000 | 464 | 0.985138004 |
MAT | numeric | 0 | 0.0000000 | 463 | 0.983014862 |
NDVI | numeric | 0 | 0.0000000 | 464 | 0.985138004 |
SiltClay | numeric | 0 | 0.0000000 | 462 | 0.980891720 |
NLCD | character | 0 | 0.0000000 | 4 | 0.008492569 |
FRG | character | 0 | 0.0000000 | 6 | 0.012738854 |
Missing Value(NA) : Variables with many missing values, i.e. those with a missing_percent close to 100, should be excluded from the analysis.
Unique value : Variables with a unique value (unique_count = 1) are considered to be excluded from data analysis. And if the data type is not numeric (integer, numeric) and the number of unique values is equal to the number of observations (unique_rate = 1), then the variable is likely to be an identifier. Therefore, this variable is also not suitable for the analysis model.
We may use diagnose_numeric(), diagnoses numeric(continuous and discrete) variables in a data frame returns more diagnostic information such as:
min : minimum value
Q1 : 1/4 quartile, 25th percentile
mean : arithmetic mean
median : median, 50th percentile
Q3 : 3/4 quartile, 75th percentile
max : maximum value
zero : number of observations with a value of 0
minus : number of observations with negative numbers
outlier : number of outliers
variables | min | Q1 | mean | median | Q3 | max | zero | minus | outlier |
---|---|---|---|---|---|---|---|---|---|
SOC | 0.4080000 | 2.7695000 | 6.3507623126 | 4.97100000 | 8.7135000 | 30.4730000 | 0 | 0 | 20 |
DEM | 258.6488037 | 1,175.3313595 | 1,631.1063060667 | 1,592.89318800 | 2,234.2648930 | 3,618.0241700 | 0 | 0 | 0 |
Slope | 0.6492527 | 1.4506671 | 4.8267398902 | 2.72667742 | 7.1070788 | 26.1041622 | 0 | 0 | 20 |
Aspect | 86.8945694 | 148.8052292 | 165.4676589153 | 164.07072450 | 179.0842895 | 255.8335266 | 0 | 0 | 8 |
TPI | -26.7086506 | -0.8160543 | -0.0006690991 | -0.04758827 | 0.8490718 | 16.7062569 | 0 | 241 | 85 |
KFactor | 0.0500000 | 0.1933357 | 0.2558965090 | 0.28000000 | 0.3200000 | 0.4300000 | 0 | 0 | 0 |
MAP | 193.9132233 | 352.7745056 | 499.3729530231 | 432.63040160 | 590.4269104 | 1,128.1145020 | 0 | 0 | 17 |
MAT | -0.5910638 | 5.8800533 | 8.8855210982 | 9.17283535 | 12.4442859 | 16.8742866 | 0 | 6 | 0 |
NDVI | 0.1424335 | 0.3053468 | 0.4354311144 | 0.41568252 | 0.5559025 | 0.7969922 | 0 | 0 | 0 |
SiltClay | 9.1619568 | 42.7587299 | 53.6779156034 | 52.11276627 | 62.8508625 | 89.8344116 | 0 | 0 | 3 |
diagnose_category() diagnoses the categorical(factor, ordered, character) variables of a data frame. The usage is similar to diagnose() but returns more diagnostic information such as:
variables : variable names
levels: level names
N : number of observation
freq : number of observation at the levels
ratio : percentage of observation at the levels
rank : rank of occupancy ratio of levels
variables | levels | N | freq | ratio | rank |
---|---|---|---|---|---|
STATE | Colorado | 471 | 136 | 28.874735 | 1 |
STATE | Wyoming | 471 | 120 | 25.477707 | 2 |
STATE | New Mexico | 471 | 109 | 23.142251 | 3 |
STATE | Kansas | 471 | 106 | 22.505308 | 4 |
NLCD | Herbaceous | 471 | 151 | 32.059448 | 1 |
NLCD | Shrubland | 471 | 130 | 27.600849 | 2 |
NLCD | Planted/Cultivated | 471 | 97 | 20.594480 | 3 |
NLCD | Forest | 471 | 93 | 19.745223 | 4 |
FRG | Fire Regime Group II | 471 | 252 | 53.503185 | 1 |
FRG | Fire Regime Group III | 471 | 100 | 21.231423 | 2 |
FRG | Fire Regime Group IV | 471 | 75 | 15.923567 | 3 |
FRG | Fire Regime Group I | 471 | 19 | 4.033970 | 4 |
FRG | Fire Regime Group V | 471 | 18 | 3.821656 | 5 |
FRG | Indeterminate FRG | 471 | 7 | 1.486200 | 6 |
diagnose_outlier() diagnoses the outliers of the numeric (continuous and discrete) variables of the data frame.
outliers_cnt : number of outliers
outliers_ratio : percent of outliers
outliers_mean : arithmetic average of outliers
with_mean : arithmetic average of with outliers
without_mean : arithmetic average of without outliers
The diagnose_outlier() produces outlier information for diagnosing the quality of the numerical data.
# A tibble: 10 × 6
variables outliers_cnt outliers_ratio outliers_mean with_mean without_mean
<chr> <int> <dbl> <dbl> <dbl> <dbl>
1 SOC 20 4.25 21.1 6.35 5.69
2 DEM 0 0 NaN 1631. 1631.
3 Slope 20 4.25 18.9 4.83 4.20
4 Aspect 8 1.70 224. 165. 164.
5 TPI 85 18.0 0.291 -0.000669 -0.0649
6 KFactor 0 0 NaN 0.256 0.256
7 MAP 17 3.61 1049. 499. 479.
8 MAT 0 0 NaN 8.89 8.89
9 NDVI 0 0 NaN 0.435 0.435
10 SiltClay 3 0.637 9.71 53.7 54.0
plot_outlier() visualizes outliers of numerical variables(continuous and discrete) of data.frame. Usage is the same diagnose().
The plot derived from the numerical data diagnosis is as follows.
With outliers box plot
Without outliers box plot
With outliers histogram
Without outliers histogram
The following example uses plot_outlier() after diagnose_outlier, and filter and select with dplyr packages to visualize this with an outlier ratio of 0.5% or higher.
normality() function of dlookr performs a normality test on multiple numerical data. Shapiro-Wilk normality test is performed. When the number of observations is greater than 5000, it is tested after extracting 5000 samples by random simple sampling.
The variables of tbl_df object returned by normality() are as follows.
statistic : Statistics of the Shapiro-Wilk test
p_value : p-value of the Shapiro-Wilk test
sample : Number of sample observations performed Shapiro-Wilk test
vars | statistic | p_value | sample |
---|---|---|---|
SOC | 0.8723726 | 0.0000000000000000003914388 | 471 |
MAP | 0.8970027 | 0.0000000000000000287526353 | 471 |
NDVI | 0.9698609 | 0.0000000291124642567419318 | 471 |
DEM | 0.9731601 | 0.0000001328862497056715322 | 471 |
MAT | 0.9732952 | 0.0000001417229160828437308 | 471 |
The normality() function supports the group_by() function syntax in the dplyr package.
variable | NLCD | statistic | p_value | sample |
---|---|---|---|---|
SOC | Planted/Cultivated | 0.9693901 | 0.02290858185622282 | 97 |
SOC | Forest | 0.9264632 | 0.00005866058197987 | 93 |
SOC | Herbaceous | 0.8892600 | 0.00000000342072181 | 151 |
SOC | Shrubland | 0.8207045 | 0.00000000003809702 | 130 |
We may also use plot_normality() function of dlookr package to visualizes the normality of numeric data. The information that plot_normality() visualizes is as follows.
Histogram of original data
Q-Q plot of original data
histogram of log transformed data
Histogram of square root transformed data
The describe() function from dloookr package computes descriptive statistics for numerical data. The descriptive statistics help determine the distribution of numerical variables.
The variables of the tbl_df object returned by describe() are as follows.
n : number of observations excluding missing values
na : number of missing values
mean : arithmetic average
sd : standard deviation
se_mean : standard error mean. sd/sqrt(n)
IQR : interquartile range (Q3-Q1)
skewness : skewness
kurtosis : kurtosis
p25 : Q1. 25% percentile
p50 : median. 50% percentile
p75 : Q3. 75% percentile
p01, p05, p10, p20, p30 : 1%, 5%, 20%, 30% percentiles
p40, p60, p70, p80 : 40%, 60%, 70%, 80% percentiles
p90, p95, p99, p100 : 90%, 95%, 99%, 100% percentiles
described_variables | n | na | mean | sd | se_mean | IQR | skewness | kurtosis | p00 | p01 | p05 | p10 | p20 | p25 | p30 | p40 | p50 | p60 | p70 | p75 | p80 | p90 | p95 | p99 | p100 |
---|---|---|---|---|---|---|---|---|---|---|---|---|---|---|---|---|---|---|---|---|---|---|---|---|---|
SOC | 467 | 4 | 6.3507623 | 5.0454091 | 0.233473691 | 5.9440000 | 1.46472837 | 2.4271923 | 0.4080000 | 0.4909400 | 0.9637000 | 1.2902000 | 2.3294000 | 2.7695000 | 3.1114000 | 3.9906000 | 4.9710000 | 6.1266000 | 7.5030000 | 8.7135000 | 10.0522000 | 13.3830000 | 16.5219000 | 22.1247600 | 30.4730000 |
DEM | 471 | 0 | 1,631.1063061 | 767.6923254 | 35.373395140 | 1,058.9335335 | -0.02350235 | -0.8039161 | 258.6488037 | 288.9806610 | 353.7696533 | 441.8609924 | 925.0328369 | 1,175.3313595 | 1,271.5794680 | 1,400.3239750 | 1,592.8931880 | 1,876.8692630 | 2,164.8010250 | 2,234.2648930 | 2,334.0241700 | 2,620.1455080 | 2,797.0867920 | 3,157.0538086 | 3,618.0241700 |
MAP | 471 | 0 | 499.3729530 | 206.9359198 | 9.535103866 | 237.6524048 | 1.08253930 | 0.4698226 | 193.9132233 | 205.0283020 | 261.5091248 | 290.6307068 | 340.8447266 | 352.7745056 | 371.9525452 | 404.3808899 | 432.6304016 | 471.3896484 | 557.4978027 | 590.4269104 | 663.0267944 | 835.6693726 | 927.7701416 | 1,102.3618041 | 1,128.1145020 |
MAT | 471 | 0 | 8.8855211 | 4.0981336 | 0.188832030 | 6.5642326 | -0.27522458 | -0.8236567 | -0.5910638 | -0.1158469 | 1.6258606 | 2.9455154 | 5.0193229 | 5.8800533 | 6.8264499 | 7.5748353 | 9.1728353 | 10.5665998 | 11.8026342 | 12.4442859 | 12.7931766 | 13.9399471 | 14.6389923 | 16.2291578 | 16.8742866 |
NDVI | 471 | 0 | 0.4354311 | 0.1620239 | 0.007465669 | 0.2505557 | 0.23375088 | -0.9180418 | 0.1424335 | 0.1631678 | 0.1920738 | 0.2215059 | 0.2756113 | 0.3053468 | 0.3317074 | 0.3769788 | 0.4156825 | 0.4773465 | 0.5348566 | 0.5559025 | 0.5853671 | 0.6759516 | 0.7216224 | 0.7601239 | 0.7969922 |
The describe() function supports the group_by() function syntax of the dplyr package. Following function calculate descriptive testatrices of SOC and NDVI of different NLCD
described_variables | NLCD | n | na | mean | sd | se_mean | IQR | skewness | kurtosis | p00 | p01 | p05 | p10 | p20 | p25 | p30 | p40 | p50 | p60 | p70 | p75 | p80 | p90 | p95 | p99 | p100 |
---|---|---|---|---|---|---|---|---|---|---|---|---|---|---|---|---|---|---|---|---|---|---|---|---|---|---|
NDVI | Forest | 93 | 0 | 0.5705648 | 0.1155016 | 0.01197696 | 0.1165678 | -0.6719584 | 0.1157272 | 0.2830779 | 0.2856635 | 0.3427034 | 0.3610759 | 0.5045352 | 0.5326702 | 0.5390721 | 0.5576768 | 0.5759758 | 0.6085463 | 0.6290170 | 0.6492380 | 0.6708930 | 0.7010803 | 0.7353695 | 0.7711932 | 0.7814745 |
NDVI | Herbaceous | 151 | 0 | 0.4003131 | 0.1307054 | 0.01063666 | 0.1257634 | 0.9764992 | 0.4084170 | 0.1648289 | 0.1785699 | 0.2555612 | 0.2651424 | 0.2944161 | 0.3124843 | 0.3308861 | 0.3497368 | 0.3769788 | 0.3934043 | 0.4193905 | 0.4382476 | 0.4771240 | 0.6033114 | 0.6916735 | 0.7305025 | 0.7337248 |
NDVI | Planted/Cultivated | 97 | 0 | 0.5332255 | 0.1213052 | 0.01231668 | 0.1373269 | 0.5177150 | -0.6469923 | 0.3249635 | 0.3262391 | 0.3740784 | 0.3937608 | 0.4236221 | 0.4498498 | 0.4584951 | 0.4879352 | 0.5132312 | 0.5297822 | 0.5670107 | 0.5871767 | 0.6677204 | 0.7226615 | 0.7493749 | 0.7969922 | 0.7969922 |
NDVI | Shrubland | 130 | 0 | 0.3065798 | 0.1295559 | 0.01136280 | 0.1688918 | 1.1160872 | 0.4843057 | 0.1424335 | 0.1501656 | 0.1663934 | 0.1871671 | 0.2014691 | 0.2101985 | 0.2158819 | 0.2352937 | 0.2691359 | 0.2868186 | 0.3441049 | 0.3790904 | 0.4145865 | 0.5239458 | 0.5461648 | 0.6790463 | 0.6939532 |
SOC | Forest | 93 | 0 | 10.4308817 | 6.8021471 | 0.70534979 | 10.3720000 | 0.7778065 | -0.1552659 | 1.3330000 | 1.3578400 | 2.3656000 | 3.3538000 | 4.4686000 | 4.9310000 | 5.1440000 | 6.5884000 | 8.9740000 | 11.1936000 | 13.7130000 | 15.3030000 | 16.6020000 | 20.8730000 | 22.2096000 | 28.1831200 | 30.4730000 |
SOC | Herbaceous | 150 | 1 | 5.4769667 | 3.9250913 | 0.32048236 | 4.3425000 | 1.2775572 | 1.4077233 | 0.4080000 | 0.5794500 | 1.0895000 | 1.4265000 | 2.2820000 | 2.6240000 | 3.0726000 | 3.5692000 | 4.6090000 | 5.2000000 | 6.3490000 | 6.9665000 | 8.2500000 | 11.0768000 | 13.3133500 | 17.5073200 | 18.8140000 |
SOC | Planted/Cultivated | 97 | 0 | 6.6967216 | 3.5983014 | 0.36535215 | 5.4170000 | 0.5350421 | -0.2587066 | 0.4620000 | 0.7192800 | 1.6380000 | 2.4642000 | 3.6094000 | 4.0020000 | 4.3260000 | 5.3820000 | 6.2300000 | 7.2166000 | 8.0810000 | 9.4190000 | 10.1170000 | 11.3962000 | 13.1846000 | 15.7638400 | 16.3360000 |
SOC | Shrubland | 127 | 3 | 4.1307638 | 3.7448591 | 0.33230251 | 4.3105000 | 1.7061821 | 2.9893653 | 0.4460000 | 0.4746400 | 0.6162000 | 0.8046000 | 1.1344000 | 1.3500000 | 1.6174000 | 2.4386000 | 2.9960000 | 3.7474000 | 4.9050000 | 5.6605000 | 6.2840000 | 9.0786000 | 12.2261000 | 15.8518000 | 19.0990000 |
correlate() calculates the correlation coefficient of all combinations of several numerical variables as follows:
var1 | var2 | coef_corr |
---|---|---|
DEM | SOC | 0.16668949 |
MAP | SOC | 0.49886194 |
MAT | SOC | -0.35802586 |
NDVI | SOC | 0.58704521 |
SOC | DEM | 0.16668949 |
MAP | DEM | -0.30672789 |
MAT | DEM | -0.80753239 |
NDVI | DEM | -0.06733319 |
SOC | MAP | 0.49886194 |
DEM | MAP | -0.30672789 |
MAT | MAP | 0.06032649 |
NDVI | MAP | 0.80528269 |
SOC | MAT | -0.35802586 |
DEM | MAT | -0.80753239 |
MAP | MAT | 0.06032649 |
NDVI | MAT | -0.20967449 |
SOC | NDVI | 0.58704521 |
DEM | NDVI | -0.06733319 |
MAP | NDVI | 0.80528269 |
MAT | NDVI | -0.20967449 |
The correlate() also supports the group_by() function syntax in the dplyr package.
NLCD | var1 | var2 | coef_corr |
---|---|---|---|
Forest | DEM | SOC | 0.30298598 |
Forest | MAP | SOC | 0.48134776 |
Forest | MAT | SOC | -0.46258320 |
Forest | NDVI | SOC | 0.39140504 |
Forest | SOC | DEM | 0.30298598 |
Forest | MAP | DEM | 0.41052474 |
Forest | MAT | DEM | -0.71735792 |
Forest | NDVI | DEM | 0.39660074 |
Forest | SOC | MAP | 0.48134776 |
Forest | DEM | MAP | 0.41052474 |
Forest | MAT | MAP | -0.62794270 |
Forest | NDVI | MAP | 0.63598331 |
Forest | SOC | MAT | -0.46258320 |
Forest | DEM | MAT | -0.71735792 |
Forest | MAP | MAT | -0.62794270 |
Forest | NDVI | MAT | -0.48149700 |
Forest | SOC | NDVI | 0.39140504 |
Forest | DEM | NDVI | 0.39660074 |
Forest | MAP | NDVI | 0.63598331 |
Forest | MAT | NDVI | -0.48149700 |
Herbaceous | DEM | SOC | -0.22121181 |
Herbaceous | MAP | SOC | 0.48276882 |
Herbaceous | MAT | SOC | -0.10104174 |
Herbaceous | NDVI | SOC | 0.52751680 |
Herbaceous | SOC | DEM | -0.22121181 |
Herbaceous | MAP | DEM | -0.57025818 |
Herbaceous | MAT | DEM | -0.66962468 |
Herbaceous | NDVI | DEM | -0.59258641 |
Herbaceous | SOC | MAP | 0.48276882 |
Herbaceous | DEM | MAP | -0.57025818 |
Herbaceous | MAT | MAP | 0.35544711 |
Herbaceous | NDVI | MAP | 0.87783385 |
Herbaceous | SOC | MAT | -0.10104174 |
Herbaceous | DEM | MAT | -0.66962468 |
Herbaceous | MAP | MAT | 0.35544711 |
Herbaceous | NDVI | MAT | 0.18936050 |
Herbaceous | SOC | NDVI | 0.52751680 |
Herbaceous | DEM | NDVI | -0.59258641 |
Herbaceous | MAP | NDVI | 0.87783385 |
Herbaceous | MAT | NDVI | 0.18936050 |
Planted/Cultivated | DEM | SOC | -0.31880666 |
Planted/Cultivated | MAP | SOC | 0.42971838 |
Planted/Cultivated | MAT | SOC | 0.06276231 |
Planted/Cultivated | NDVI | SOC | 0.47472055 |
Planted/Cultivated | SOC | DEM | -0.31880666 |
Planted/Cultivated | MAP | DEM | -0.88745536 |
Planted/Cultivated | MAT | DEM | -0.83305530 |
Planted/Cultivated | NDVI | DEM | -0.52616146 |
Planted/Cultivated | SOC | MAP | 0.42971838 |
Planted/Cultivated | DEM | MAP | -0.88745536 |
Planted/Cultivated | MAT | MAP | 0.62195665 |
Planted/Cultivated | NDVI | MAP | 0.71441683 |
Planted/Cultivated | SOC | MAT | 0.06276231 |
Planted/Cultivated | DEM | MAT | -0.83305530 |
Planted/Cultivated | MAP | MAT | 0.62195665 |
Planted/Cultivated | NDVI | MAT | 0.17053661 |
Planted/Cultivated | SOC | NDVI | 0.47472055 |
Planted/Cultivated | DEM | NDVI | -0.52616146 |
Planted/Cultivated | MAP | NDVI | 0.71441683 |
Planted/Cultivated | MAT | NDVI | 0.17053661 |
Shrubland | DEM | SOC | 0.39720120 |
Shrubland | MAP | SOC | 0.44532165 |
Shrubland | MAT | SOC | -0.45936785 |
Shrubland | NDVI | SOC | 0.64602818 |
Shrubland | SOC | DEM | 0.39720120 |
Shrubland | MAP | DEM | 0.29834522 |
Shrubland | MAT | DEM | -0.81913433 |
Shrubland | NDVI | DEM | 0.48180780 |
Shrubland | SOC | MAP | 0.44532165 |
Shrubland | DEM | MAP | 0.29834522 |
Shrubland | MAT | MAP | -0.28844730 |
Shrubland | NDVI | MAP | 0.70639285 |
Shrubland | SOC | MAT | -0.45936785 |
Shrubland | DEM | MAT | -0.81913433 |
Shrubland | MAP | MAT | -0.28844730 |
Shrubland | NDVI | MAT | -0.57829256 |
Shrubland | SOC | NDVI | 0.64602818 |
Shrubland | DEM | NDVI | 0.48180780 |
Shrubland | MAP | NDVI | 0.70639285 |
Shrubland | MAT | NDVI | -0.57829256 |
plot.correlate() visualizes the correlation matrix.
The plot.correlate() function also supports the group_by() function syntax in the dplyr package.
Create a R-Markdown documents (name homework_04.rmd) in this project and do all Tasks ( using the data shown below.
Submit all codes and output as a HTML document (homework_04.html) before class of next week.
tidyverse and dlookr.
Download the data and save in your project directory. Use read_csv to load the data in your R-session. For example:
mf<-read_csv(“bd_arsenic_data_raw.csv”))
Use dlookr::diagnose() function of dlookr package to do Data Quality Diagnosis all variables
Use dlookr::diagnose_numeric(), diagnoses numeric such as GAs,StAs, WAs, SAs, SPAs,SAoAs and SAoFe
Use dlookr::diagnose_category() to diagnoses the categorical variables (Land_type and variety) of a data frame.
Use dlookr::diagnose_outlier() to diagnosis outlier of GAs, StAs, WAs, and SAs.
Use dlookr::plot_outlier() to visualizes outliers of SAs
Performs a normality test on multiple numerical data (such as GAs,StAs, WAs,SAs, SPAs,SAoAs and SAoFe) using dlookr::normality() function, .
Use dlookr::normality() and dplyr::group_by() check normality of rice garin As (GAs) in different rice varieties.
Plot histogram and QQ-plot of SAs and histogram and square root transformed SAs using dlookr::plot_normality() function
Create a descriptive statistics table for GAs, StAs, Soil, WAs, PAs using dlookr::describe() and flextable() functions
Use dlookr::describe() and dplyr::group_by() functions calculate descriptive testatrices of SAs, SPAs, SAoAs and SAoFe in different landtypes
Calculates the correlation coefficient of all combinations of WAs, WFe, WP, WEc, SEc, SAs, SPAs, SAoAs and SAoFe and plot them using dlookr::correlate() and plot() functions.
Calculates the correlation coefficients in two landtypes: use dlookr::correlate() and dplyr::group_by()
# Data Exploration with dlookr {.unnumbered}
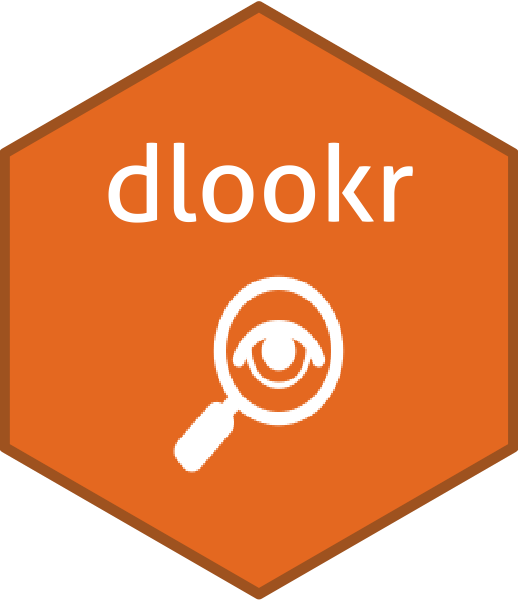{width="97"}
The dlookr is a collection of tools that support data diagnosis, exploration, and transformation. Data diagnostics provides information and visualization of missing values and outliers and unique and negative values to help you understand the distribution and quality of your data. Data exploration provides information and visualization of the descriptive statistics of univariate variables, normality tests and outliers, correlation of two variables, and relationship between target variable and predictor. Data transformation supports binning for categorizing continuous variables, imputates missing values and outliers, resolving skewness. And it creates automated reports that support these three tasks.
Features:
- Diagnose data quality.
- Find appropriate scenarios to pursuit the follow-up analysis through data exploration and understanding.
- Derive new variables or perform variable transformations.
- Automatically generate reports for the above three tasks.
- Supports quality diagnosis and EDA of table of DBMS
#### Usage
- Data quality diagnosis for data.frame, tbl_df, and table of DBMS
- Exploratory Data Analysis for data.frame, tbl_df, and table of DBMS
- Data Transformation
- Data diagnosis and EDA for table of DBMS
> install.packages("dlookr")
### Load Library
```{r Load Library, message=FALSE, warning=FALSE}
library(dlookr)
```
### Data
In this exercise we will use following data set. The data set has missing values.
[gp_soil_data_na.csv](https://www.dropbox.com/s/9ikm5yct36oflei/gp_soil_data.csv?dl=0)
```{r}
#| warning: false
#| error: false
library(tidyverse)
# define file from my github
urlfile = "https://github.com/zia207/r-colab/raw/main/Data/USA/gp_soil_data_na.csv"
mf<-read_csv(url(urlfile))
```
### Data Quality Diagnosis
#### General diagnosis of all variables
Data Quality Diagnosis is the first step before any statistical analysis. We use **diagnose()** function of **dlookr** package to do general General diagnosis of all variables.
The variables of the tbl_df object returned by diagnose () are as follows:
- variables : variable names
- types : the data type of the variables
- missing_count : number of missing values
- missing_percent : percentage of missing values
- unique_count : number of unique values
- unique_rate : rate of unique value. unique_count / number of observation
```{r}
#| warning: false
#| error: false
library (flextable)
dlookr::diagnose(mf)%>%
flextable()
```
Missing Value(NA) : Variables with many missing values, i.e. those with a missing_percent close to 100, should be excluded from the analysis.
Unique value : Variables with a unique value (unique_count = 1) are considered to be excluded from data analysis. And if the data type is not numeric (integer, numeric) and the number of unique values is equal to the number of observations (unique_rate = 1), then the variable is likely to be an identifier. Therefore, this variable is also not suitable for the analysis model.
#### Diagnosis of Numeric Variables
We may use **diagnose_numeric()**, diagnoses numeric(continuous and discrete) variables in a data frame returns more diagnostic information such as:
- min : minimum value
- Q1 : 1/4 quartile, 25th percentile
- mean : arithmetic mean
- median : median, 50th percentile
- Q3 : 3/4 quartile, 75th percentile
- max : maximum value
- zero : number of observations with a value of 0
- minus : number of observations with negative numbers
- outlier : number of outliers
```{r}
#| warning: false
#| error: false
# First select numerical columns
mf %>%
dplyr::select(SOC, DEM, Slope, Aspect, TPI, KFactor, MAP, MAT, NDVI, SiltClay) %>%
# then diagnose them
dlookr::diagnose_numeric()%>%
flextable()
```
#### Diagnosis of Categorical Variables
**diagnose_category()** diagnoses the categorical(factor, ordered, character) variables of a data frame. The usage is similar to diagnose() but returns more diagnostic information such as:
- variables : variable names
- levels: level names
- N : number of observation
- freq : number of observation at the levels
- ratio : percentage of observation at the levels
- rank : rank of occupancy ratio of levels
```{r}
#| warning: false
#| error: false
mf %>%
# Select categorical variables
dplyr::select(STATE, NLCD,FRG) %>%
# then diagnose them
dlookr::diagnose_category()%>%
flextable()
```
### Diagnosing Outliers
diagnose_outlier() diagnoses the outliers of the numeric (continuous and discrete) variables of the data frame.
- outliers_cnt : number of outliers
- outliers_ratio : percent of outliers
- outliers_mean : arithmetic average of outliers
- with_mean : arithmetic average of with outliers
- without_mean : arithmetic average of without outliers
The **diagnose_outlier()** produces outlier information for diagnosing the quality of the numerical data.
```{r}
mf %>% dlookr::diagnose_outlier(SOC, DEM, SOC, Slope, Aspect, TPI, KFactor, MAP, MAT, NDVI, SiltClay)
```
#### Visualization of Outliers
**plot_outlier()** visualizes outliers of numerical variables(continuous and discrete) of data.frame. Usage is the same diagnose().
The plot derived from the numerical data diagnosis is as follows.
- With outliers box plot
- Without outliers box plot
- With outliers histogram
- Without outliers histogram
The following example uses plot_outlier() after diagnose_outlier, and filter and select with dplyr packages to visualize this with an outlier ratio of 0.5% or higher.
```{r}
#| warning: false
#| error: false
#| fig.width: 5.5
#| fig.height: 5
mf %>%
dlookr::plot_outlier(dlookr::diagnose_outlier(mf,SOC) %>%
dplyr::filter(outliers_ratio >= 0.5) %>%
dplyr::select(variables) %>%
unlist())
```
### Daignosis Normality
#### Normality Test
**normality()** function of **dlookr** performs a normality test on multiple numerical data. Shapiro-Wilk normality test is performed. When the number of observations is greater than 5000, it is tested after extracting 5000 samples by random simple sampling.
The variables of tbl_df object returned by normality() are as follows.
- statistic : Statistics of the Shapiro-Wilk test
- p_value : p-value of the Shapiro-Wilk test
- sample : Number of sample observations performed Shapiro-Wilk test
```{r }
#| warning: false
#| error: false
mf %>%
dplyr::select(SOC, DEM, MAP, MAT, NDVI) %>%
dlookr::normality() %>%
# sort variables that do not follow a normal distribution in order of p_value:
dplyr::filter(p_value <= 0.01) %>%
dplyr::arrange(abs(p_value)) %>%
flextable()
```
The normality() function supports the **group_by()** function syntax in the dplyr package.
```{r }
#| warning: false
#| error: false
mf %>%
dplyr::group_by(NLCD) %>%
dlookr::normality(SOC) %>%
dplyr:: arrange(desc(p_value)) %>%
flextable()
```
#### Visualization of Normality
We may also use **plot_normality()** function of **dlookr** package to visualizes the normality of numeric data. The information that plot_normality() visualizes is as follows.
- Histogram of original data
- Q-Q plot of original data
- histogram of log transformed data
- Histogram of square root transformed data
```{r}
#| warning: false
#| error: false
#| fig.width: 5.5
#| fig.height: 5
mf %>% dlookr::plot_normality(SOC)
```
### Descriptive Statistics
The **describe()** function from **dloookr** package computes descriptive statistics for numerical data. The descriptive statistics help determine the distribution of numerical variables.
The variables of the tbl_df object returned by describe() are as follows.
- n : number of observations excluding missing values
- na : number of missing values
- mean : arithmetic average
- sd : standard deviation
- se_mean : standard error mean. sd/sqrt(n)
- IQR : interquartile range (Q3-Q1)
- skewness : skewness
- kurtosis : kurtosis
- p25 : Q1. 25% percentile
- p50 : median. 50% percentile
- p75 : Q3. 75% percentile
- p01, p05, p10, p20, p30 : 1%, 5%, 20%, 30% percentiles
- p40, p60, p70, p80 : 40%, 60%, 70%, 80% percentiles
- p90, p95, p99, p100 : 90%, 95%, 99%, 100% percentiles
```{r}
#| warning: false
#| error: false
# First select numerical columns
des.stata<-mf %>%
dplyr::select(SOC, DEM, MAP, MAT, NDVI) %>%
# then descrive them
dlookr::describe()
flextable(des.stata)
```
The **describe()** function supports the **group_by()** function syntax of the **dplyr** package. Following function calculate descriptive testatrices of SOC and NDVI of different NLCD
```{r}
mf %>%
group_by(NLCD) %>%
dlookr::describe(SOC, NDVI) %>%
flextable()
```
### Correlation Analysis
**correlate()** calculates the correlation coefficient of all combinations of several numerical variables as follows:
```{r}
#| warning: false
#| error: false
# First select numerical columns
mf %>%
dplyr::select(SOC, DEM, MAP, MAT, NDVI) %>%
# then diagnose them
dlookr::correlate()%>%
flextable()
```
The correlate() also supports the group_by() function syntax in the dplyr package.
```{r}
#| warning: false
#| error: false
mf %>%
group_by(NLCD) %>%
dplyr::select(SOC, DEM, MAP, MAT, NDVI) %>%
# then diagnose them
dlookr::correlate()%>%
flextable()
```
### Visualization of the Correlation Matrix
**plot.correlate()** visualizes the correlation matrix.
```{r}
#| warning: false
#| error: false
#| fig.width: 5.5
#| fig.height: 5
mf %>%
dplyr::select(SOC, DEM, MAP, MAT, NDVI) %>%
# then diagnose them
dlookr::correlate()%>%
plot()
```
The plot.correlate() function also supports the group_by() function syntax in the dplyr package.
```{r}
#| warning: false
#| error: false
mf %>%
group_by(NLCD) %>%
dplyr::select(SOC, DEM, MAP, MAT, NDVI) %>%
# then diagnose them
dlookr::correlate()%>%
plot()
```
### Exercise
1. Create a R-Markdown documents (name homework_04.rmd) in this project and do all Tasks ( using the data shown below.
2. Submit all codes and output as a HTML document (homework_04.html) before class of next week.
#### Required R-Package
tidyverse and dlookr.
#### Data
[bd_arsenic_data_raw.csv](https://www.dropbox.com/s/6kw0wkprpw31kis/bd_arsenic_data_raw.csv?dl=0)
Download the data and save in your project directory. Use read_csv to load the data in your R-session. For example:
> mf\<-read_csv("bd_arsenic_data_raw.csv"))
#### Tasks
1. Use dlookr::diagnose() function of dlookr package to do Data Quality Diagnosis all variables
2. Use dlookr::diagnose_numeric(), diagnoses numeric such as GAs,StAs, WAs, SAs, SPAs,SAoAs and SAoFe
3. Use dlookr::diagnose_category() to diagnoses the categorical variables (Land_type and variety) of a data frame.
4. Use dlookr::diagnose_outlier() to diagnosis outlier of GAs, StAs, WAs, and SAs.
5. Use dlookr::plot_outlier() to visualizes outliers of SAs
6. Performs a normality test on multiple numerical data (such as GAs,StAs, WAs,SAs, SPAs,SAoAs and SAoFe) using dlookr::normality() function, .
7. Use dlookr::normality() and dplyr::group_by() check normality of rice garin As (GAs) in different rice varieties.
8. Plot histogram and QQ-plot of SAs and histogram and square root transformed SAs using dlookr::plot_normality() function
9. Create a descriptive statistics table for GAs, StAs, Soil, WAs, PAs using dlookr::describe() and flextable() functions
10. Use dlookr::describe() and dplyr::group_by() functions calculate descriptive testatrices of SAs, SPAs, SAoAs and SAoFe in different landtypes
11. Calculates the correlation coefficient of all combinations of WAs, WFe, WP, WEc, SEc, SAs, SPAs, SAoAs and SAoFe and plot them using dlookr::correlate() and plot() functions.
12. Calculates the correlation coefficients in two landtypes: use dlookr::correlate() and dplyr::group_by()
### Further Reading
1. [dlook](https://github.com/choonghyunryu/dlookr)
2. [Package dlookr](https://cran.r-project.org/web/packages/dlookr/dlookr.pdf)
3. [R package reviews-dlookr](https://yuzar-blog.netlify.app/posts/2021-01-30-r-package-reviews-dlookr-diagnose-explore-and-transform-your-data/)